About Me
I am a Ph.D. Candidate in Computer Science at Northeastern University, focusing on Video-Text Understanding. My research interests include open-world video understanding, temporal modeling, action segmentation, and weakly supervised video-text learning.
Prior to my Ph.D., I received dual B.S. degrees in Computer Science & Economics from NYU Shanghai with a Major GPA of 3.98/4. I have extensive research and industry experience, having worked as a Research Assistant with Prof. Ehsan Elhamifar, a Research Intern at Microsoft's Responsible and Open AI Research Team, an Applied Scientist Intern at Amazon's AWS AI Lab, a Student Researcher at Chinese Academic of Sciences, and an Architecture Team Summer Intern at NVIDIA.
My research aims to advance the understanding of video content through efficient temporal modeling, action segmentation, error detection, and multi-object tracking. I am actively looking for collaboration opportunities in computer vision and video understanding.
Updates
- 04-2025 My intern paper at Microsoft is accepted to CVPR 2025.
- 06-2024 Started Research Internship at Microsoft Responsible and Open AI Research Team.
- 04-2024 Three papers accepted to CVPR 2024. My intern paper as Amazon is designated as "Highlight" paper.
- 09-2022 Started as an Applied Scientist Intern at Amazon's AWS AI Lab.
- 04-2022 One papers accepted to CVPR 2022.
Selected Publications
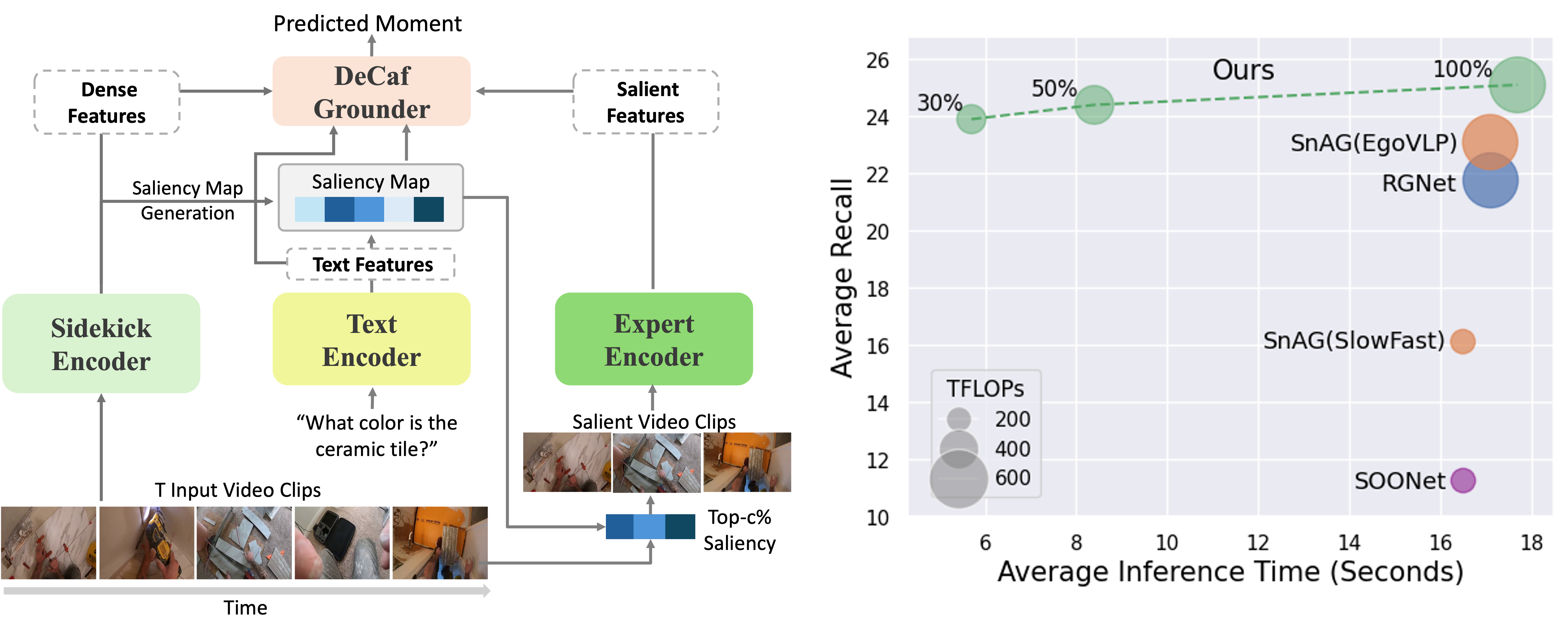
DeCafNet: Delegate and Conquer for Efficient Temporal Grounding in Long Videos
CVPR 2025
Proposed a novel Delegate-and-Conquer method that achieves state-of-the-art performance with 47%-66% lower computation for efficient temporal sentence grounding in hour-long videos.
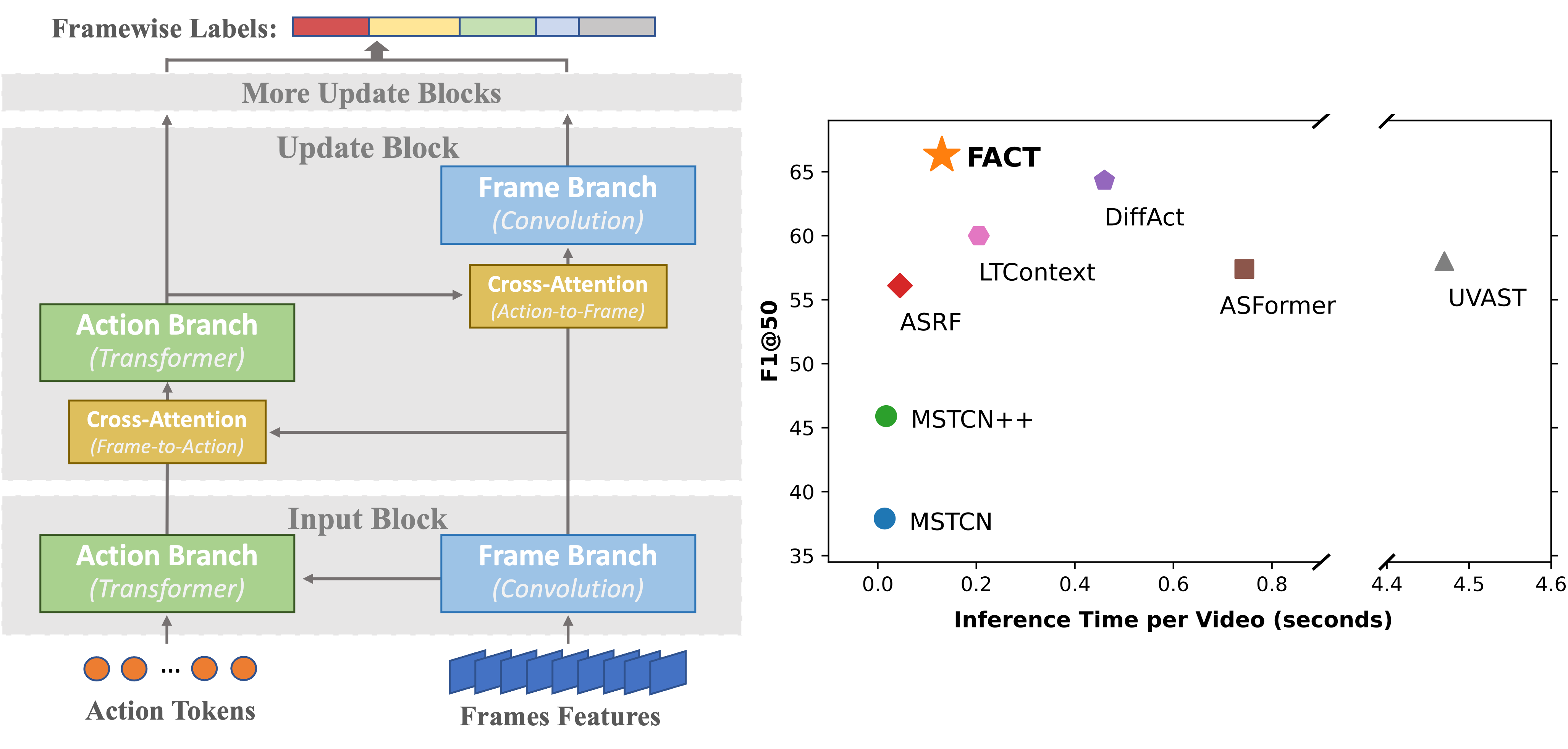
FACT: Frame-Action Cross-Attention Temporal Modeling for Efficient Fully-Supervised Action Segmentation
CVPR 2024
Proposed an efficient framework of synchronized temporal modeling on multi-levels (frame/action). Achieved state-of-the-art results on 4 datasets with lower inference time and enabled Vision-Language Learning.
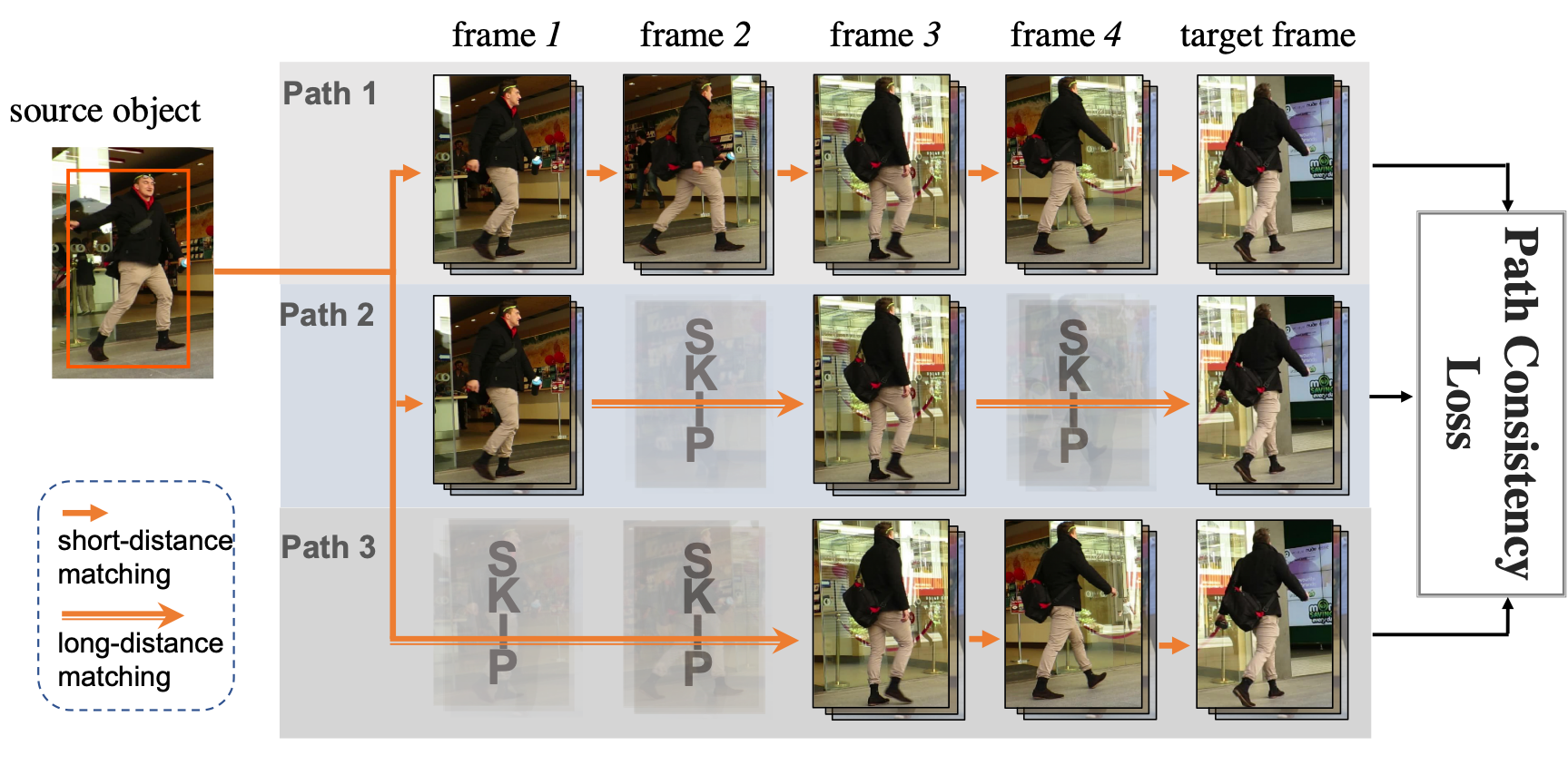
Self-Supervised Multi-Object Tracking with Path Consistency
CVPR 2024 Highlight
Proposed a new tracking self-supervision objective with improved robustness to occlusion and appearance changes. Outperformed existing works on popular benchmarks.
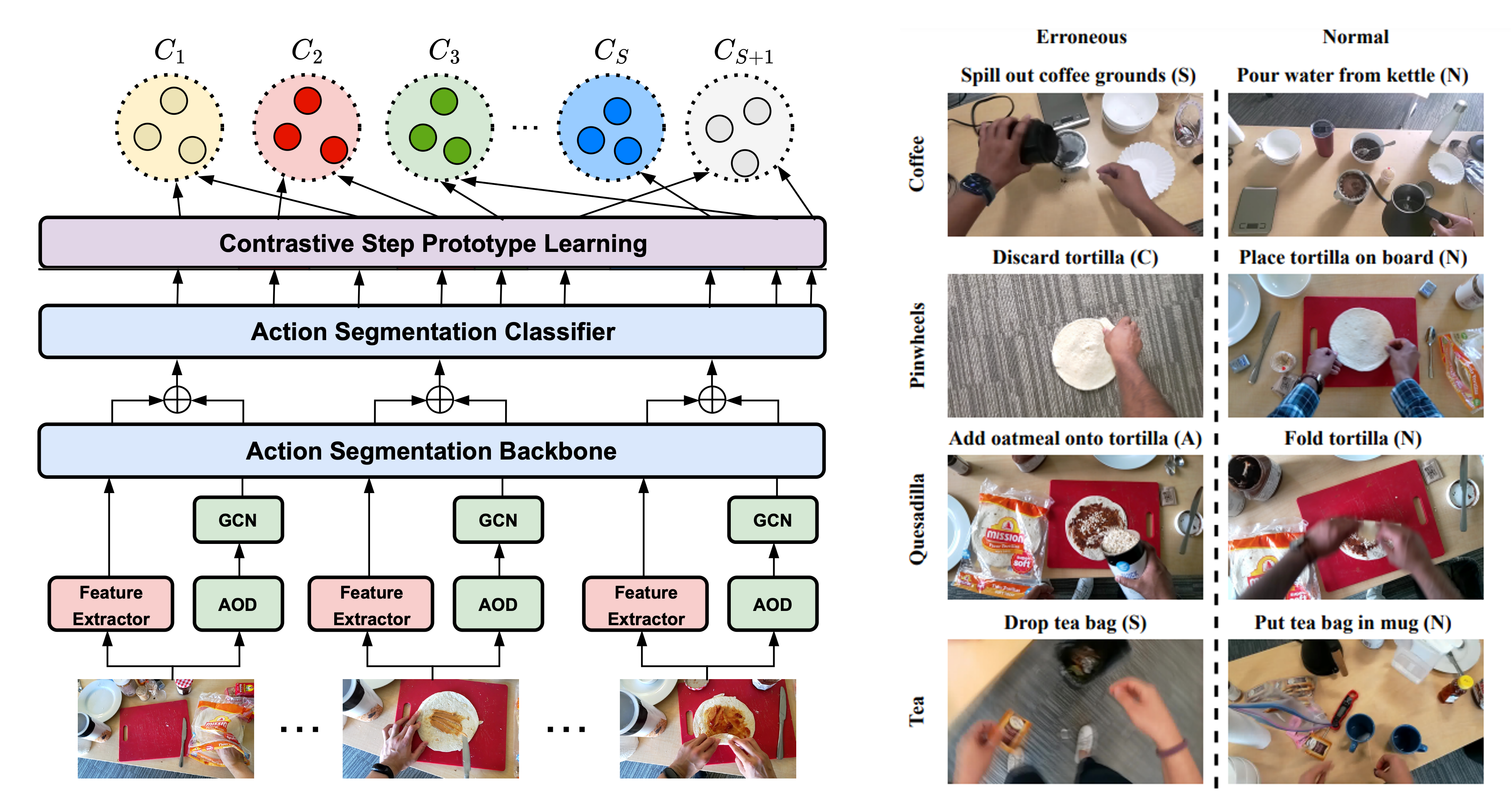
Error Detection in Egocentric Procedural Task Videos
CVPR 2024
Proposed Spatio-Temporal & Contrastive Learning method for error detection in procedural videos. Collected Egocentric Procedural Error Detection dataset of extensive error types.
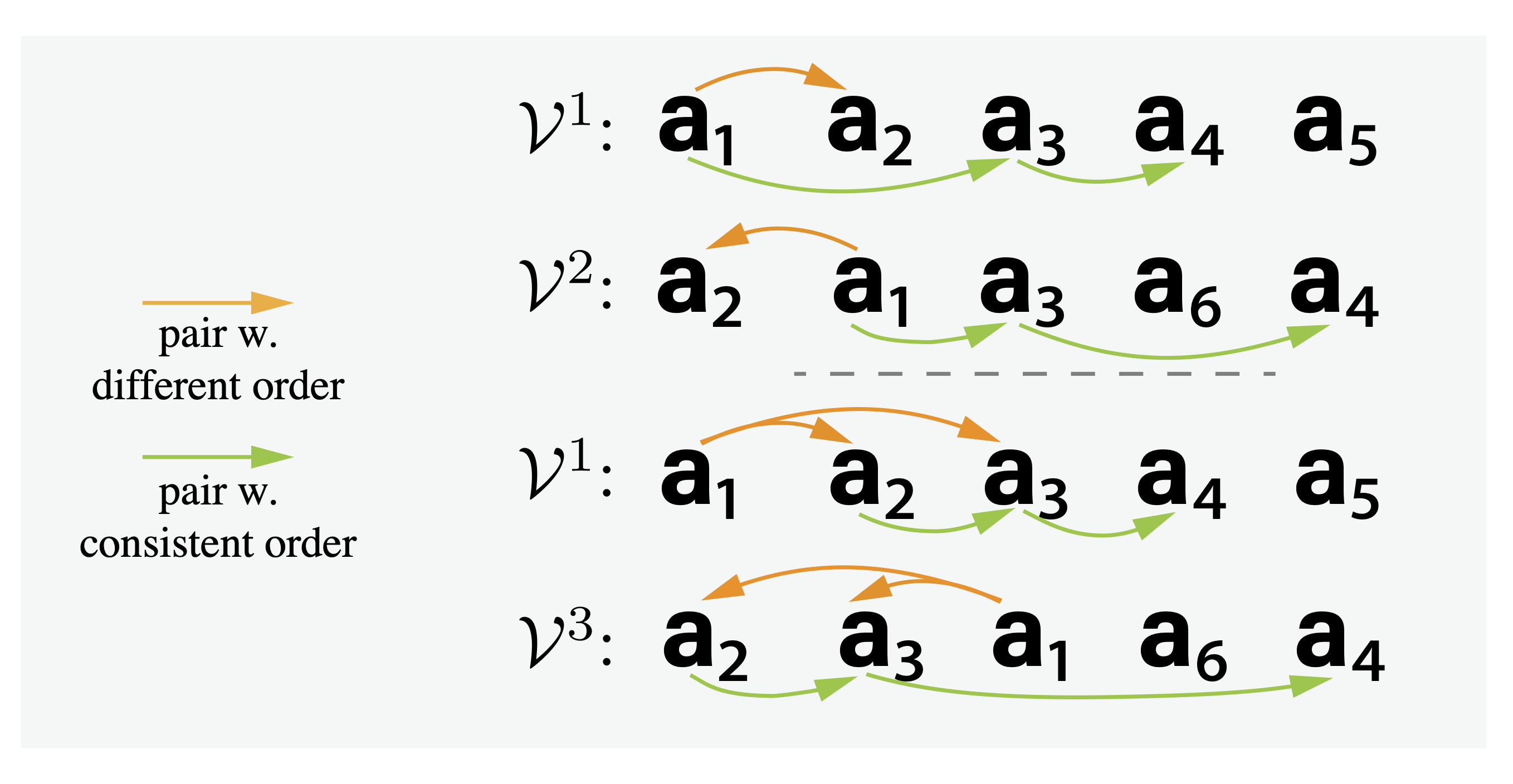
Set-Supervised Action Learning in Procedural Task Videos via Pairwise Order Consistency
CVPR 2022
Proposed enhanced Action Modeling via Multi-Dimension Subspaces to capture large intra-class variations in weakly supervised video-text learning scenarios.
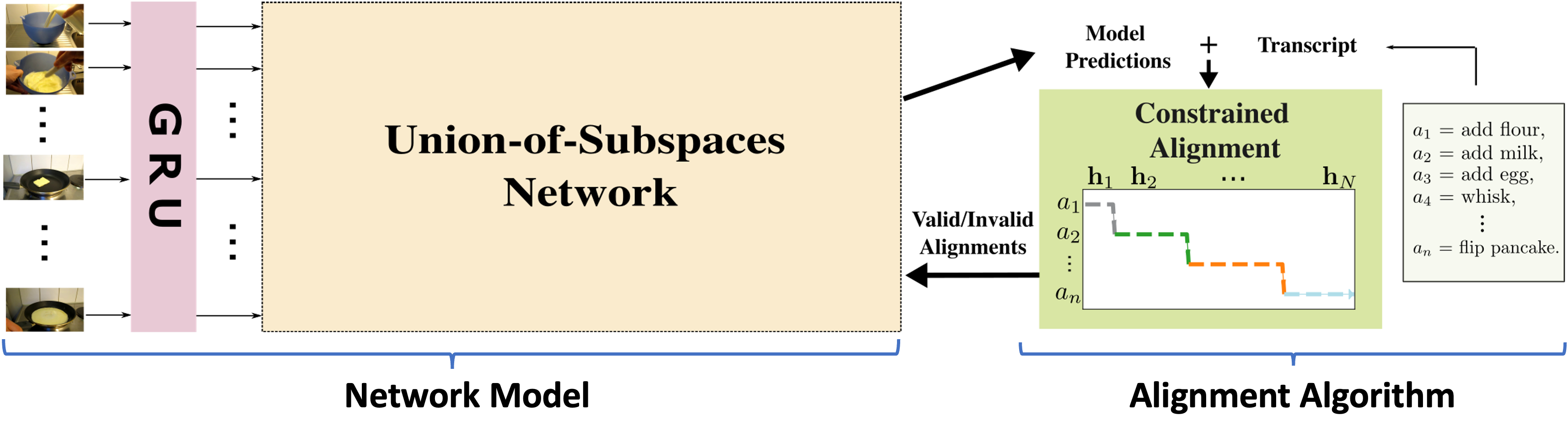
Weakly-supervised Action Segmentation and Alignment via Transcript-Aware Union-of-subspaces Learning
ICCV 2022
Proposed Self-Supervised method to Recover and Learn Action Temporal Dependencies; Doubled the accuracies of state-of-the-art approaches for weakly supervised video-text learning.
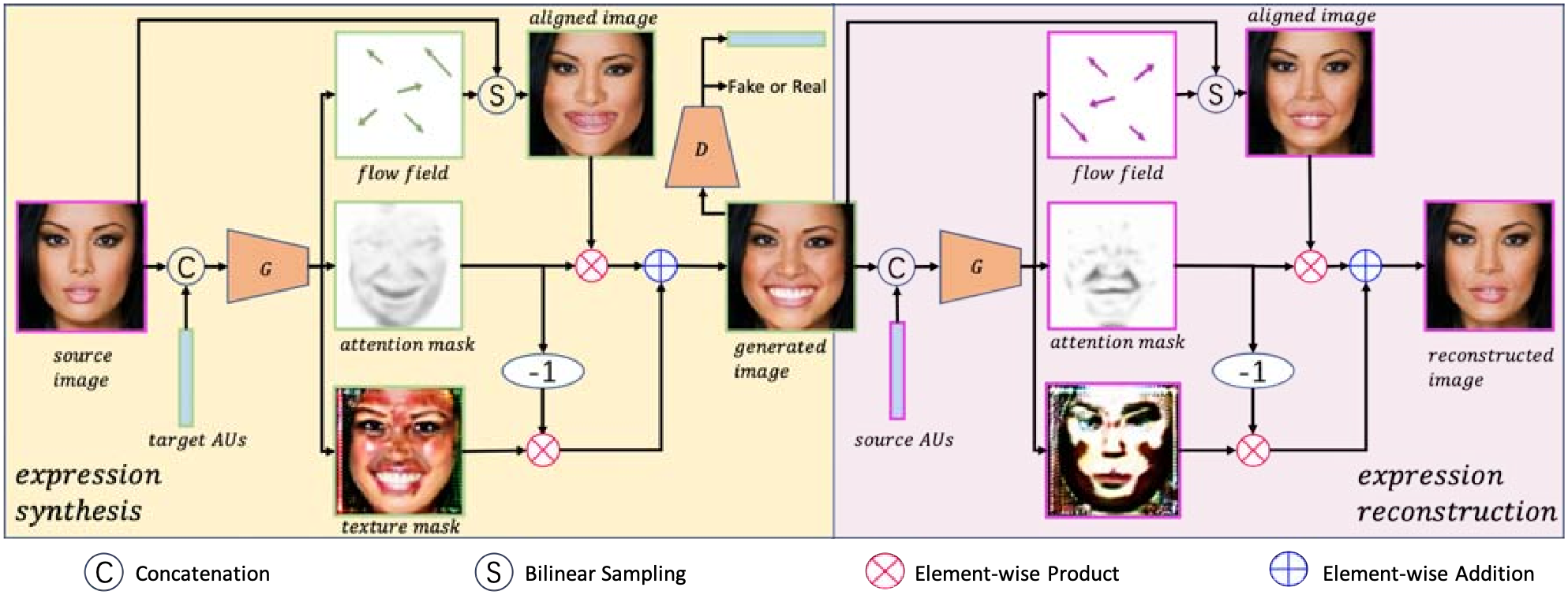
Dft-Net: Disentanglement of Face Deformation and Texture Synthesis for Expression Editing
ICIP 2019
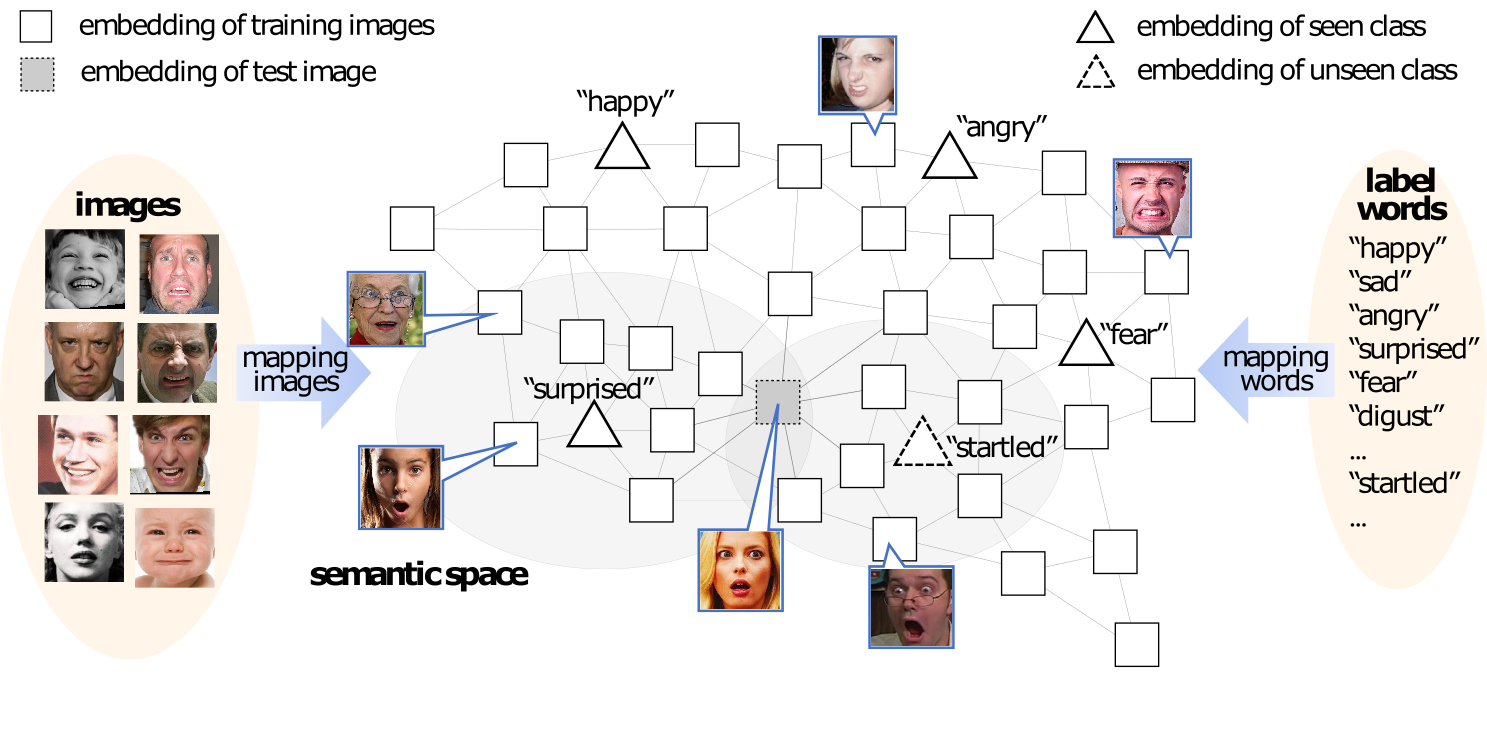
Zero-Shot Facial Expression Recognition with Multi-Label Label Propagation
ACCV 2018 Oral
Proposed a new Transductive Label Propagation method and the first Open-Set Facial Expression Recognition dataset.
Experience
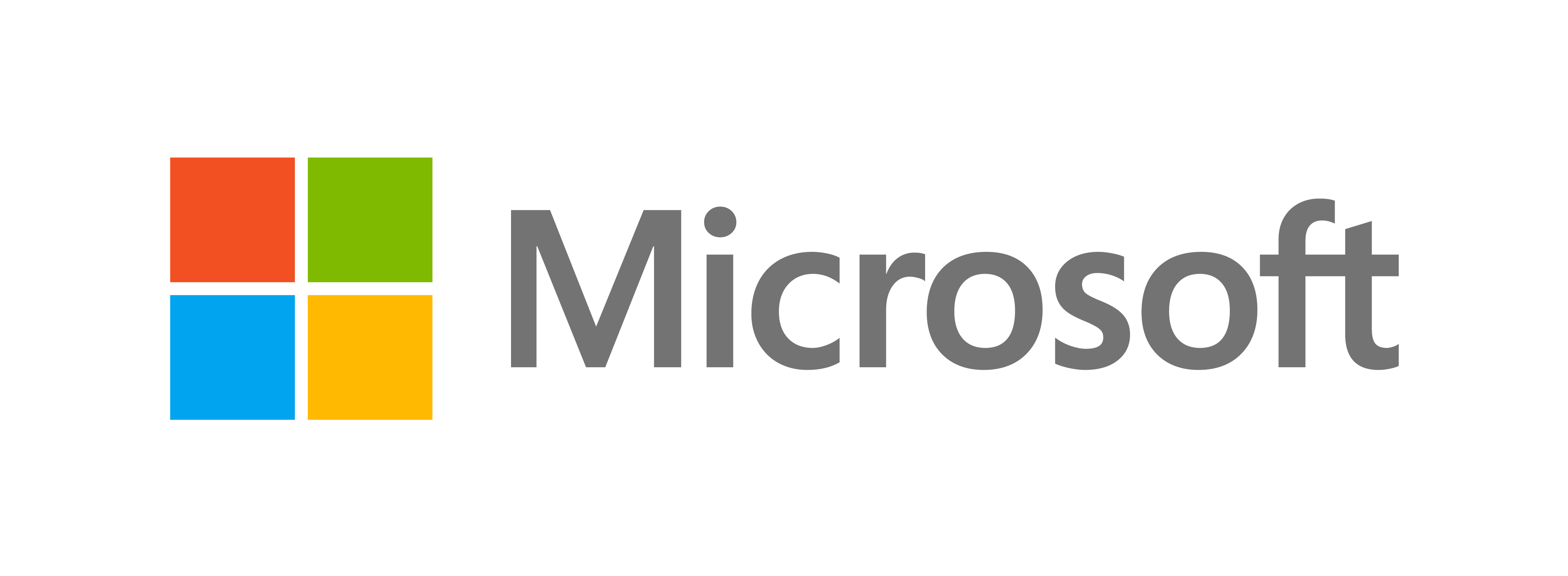
Worked on advancing responsible AI technologies with a focus on video understanding.
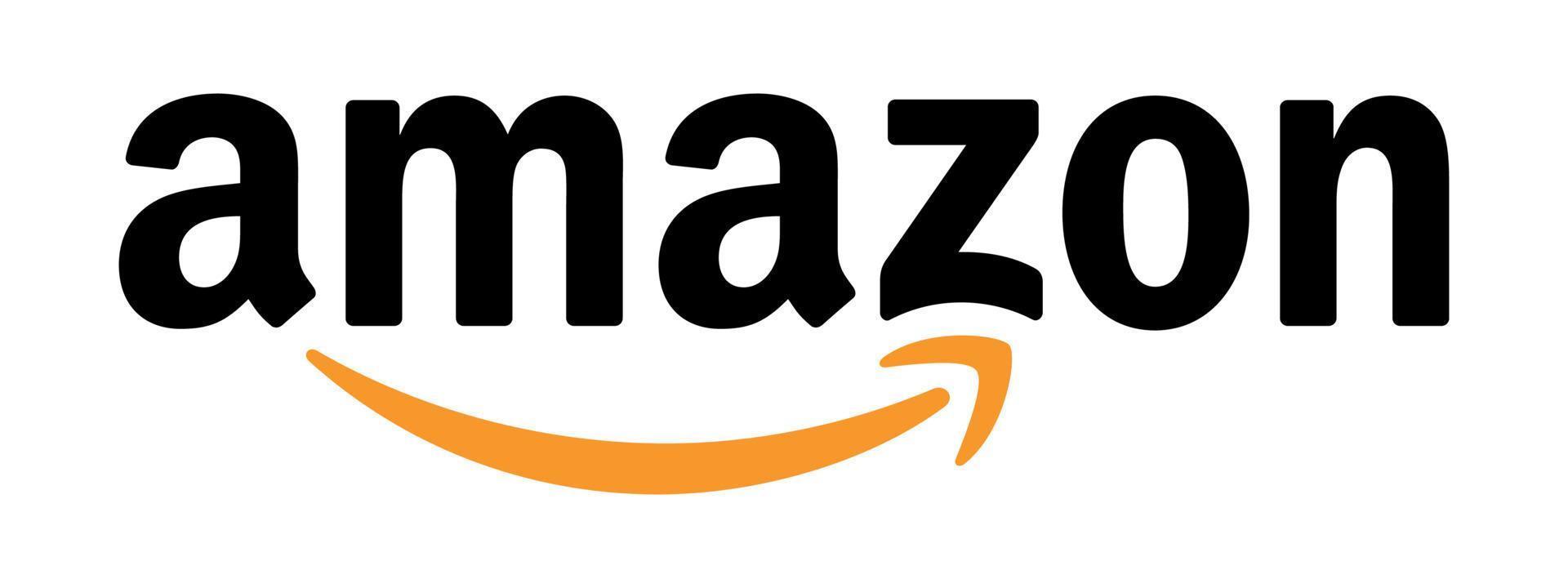
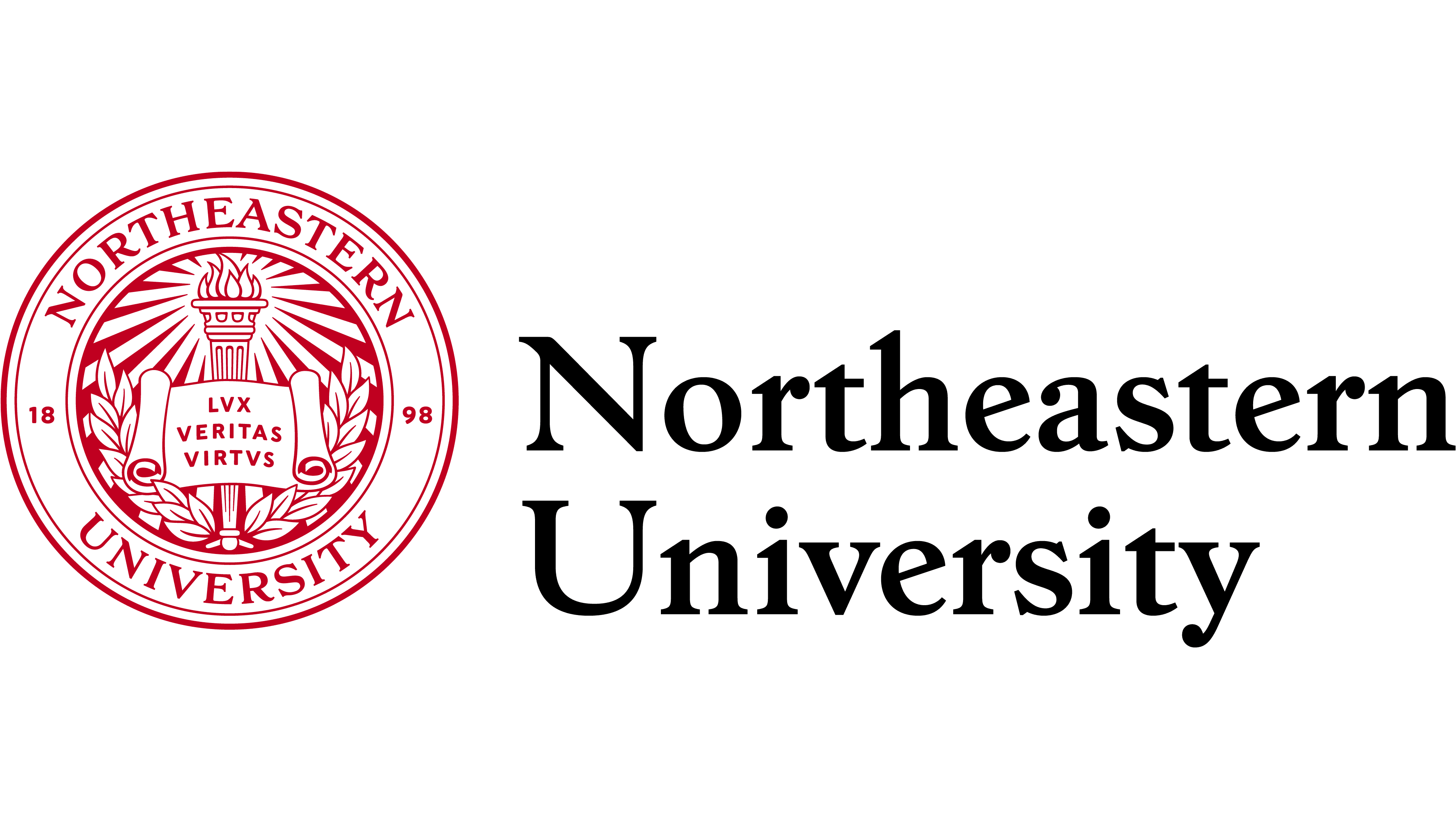
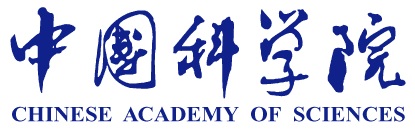
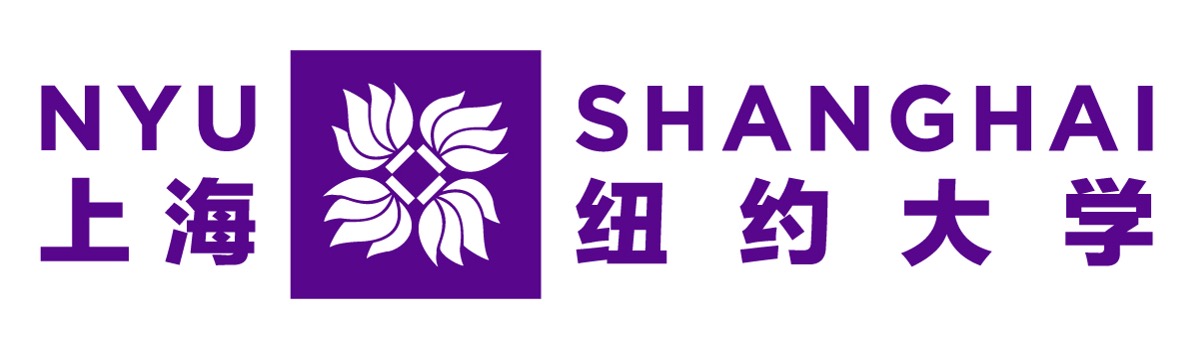
Graduated with a Major GPA of 3.98/4. Conducted research in computer vision and machine learning.